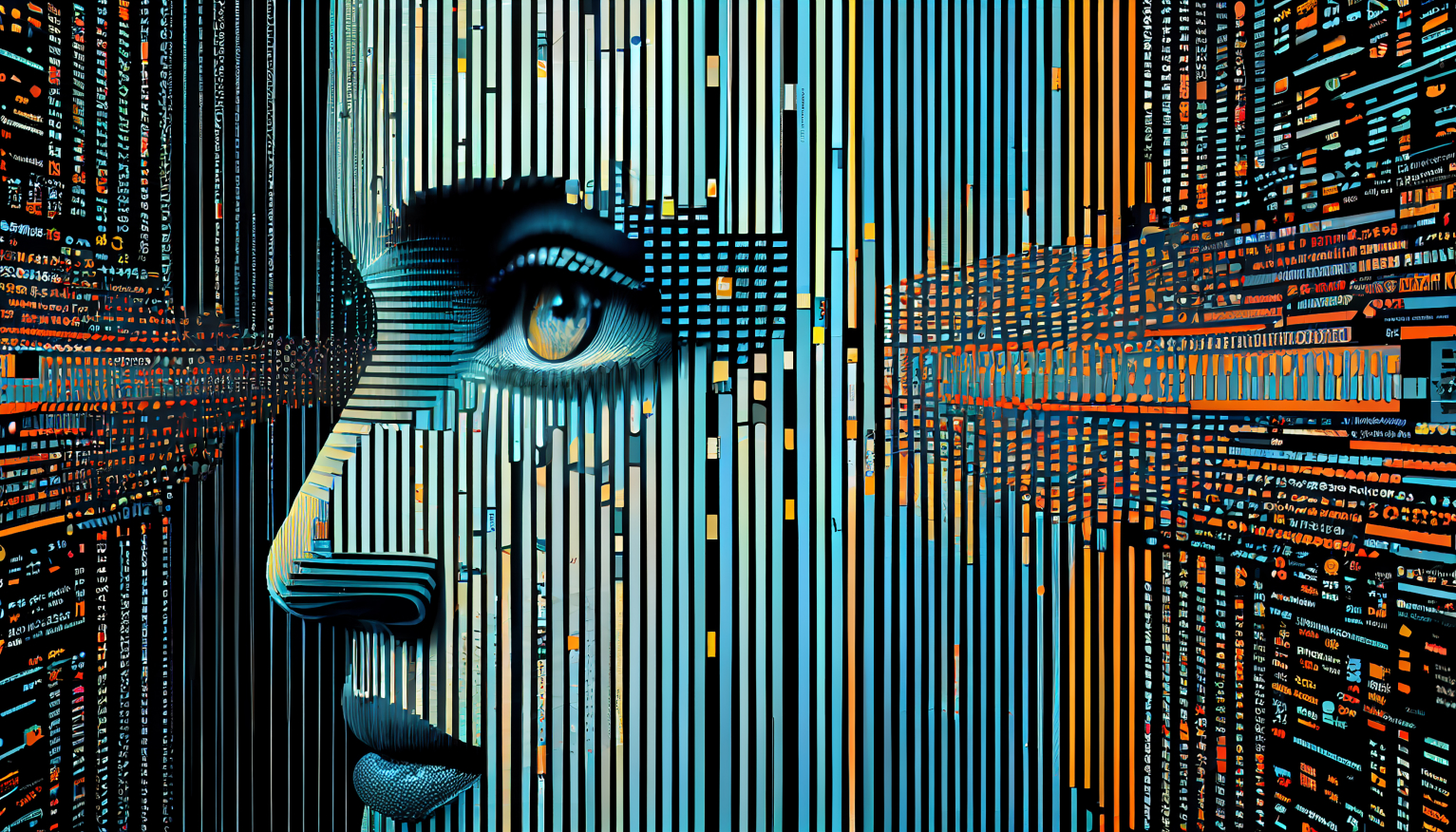
From Idea to Impact: The Six Stages of Generative AI Adoption Made Simple with Databricks

Introduction
The rapid advancements in Generative AI (Generative AI) are transforming how businesses innovate, operate, and grow. While many companies are eager to harness the potential of Generative AI, the path from exploration to business impact can be daunting. Databricks, with its unified data and AI platform, simplifies this journey, empowering organizations to move seamlessly through the six stages of Generative AI adoption.
Artificial intelligence and its various sub-sections are being democratized and becoming popular due to a growing open-source community in development of high quality of models, improvement in graphics and central processing units for faster processing of images and large datasets respectively, and great innovative strides in algorithms such as GANs and Transfomers Architecture. These strides have made adoption of artificial intelligence a necessity to being competitive as a business.
This article will introduce a real-world business case for a retail company exploring Generative AI, focusing on two high-impact use cases to illustrate how Databricks accelerates every stage of the adoption process.
The Six Stages of Generative AI Adoption
Ideation: Defining the Opportunity
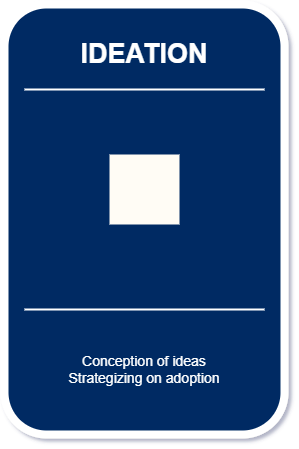
Businesses start by identifying how Generative AI can address their pain points or open new opportunities. This involves understanding of the operations of a business and what Generative AI provides.
A global retail chain which aims to leverage Generative AI can consider enhancing customer experiences and operational efficiency. In this initial stage of adoption, the business may identify four potential use cases:
- Personalized marketing campaigns
- Inventory demand forecasting
- Automated customer support through AI chatbots
- Product image generation for e-commerce
For immediate impact and and the availability of datasets for pre-training or fine-tuning models, the business may then decide to focus on the implementation of the following:
- Personalized marketing campaigns: Tailoring marketing promotions based on customer behavior to drive sales. This makes it possible to generate personalized marketing campaigns to individual customers which will better influence purchasing decisions.
- Inventory demand forecasting: Modeling patterns in sales and promotional outputs will help the business in predicting stock needs to reduce costs and optimize supply chains.
How Databricks Helps
Collaborative notebooks offered by Databricks foster brainstorming and exploration by cross-functional teams. This will make it easier for teams to perform exploratory analysis on datasets.
Built-in integration with tools like MLflow and access to pre-trained Generative AI models accelerate proof-of-concept ideas.
Data Preparation: Building a Strong Foundation
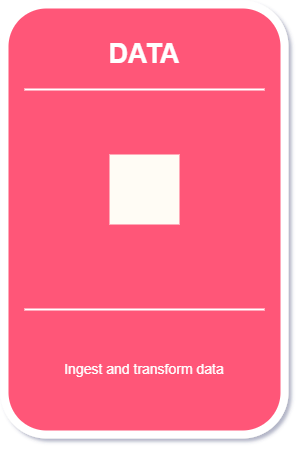
The most fundamental component to build a tailored generative AI solution for a business is access to clean and structured data. It is critical for training Generative AI models to generate content which are tailored to the needs and unique pain points of the business.
How Databricks Helps
Databricks' Lakehouse architecture unifies structured and unstructured data for seamless analysis since it leverages resilient and scalable data lakes for the storage of massive amounts of data.
Features like Delta Lake ensure high-quality, version-controlled data pipelines.
The query foundation feature of Databricks’ Unity Catalog offers prebuilt connectors for third-party data sources to simplify data ingestion.
For personalized marketing, the features elaborated will aid in consolidating customer interaction data, including purchase history, website visits, and demographics. For demand forecasting, integration of historical sales, seasonal trends, and supplier data will be seamless.
Model Development: Innovating with AI
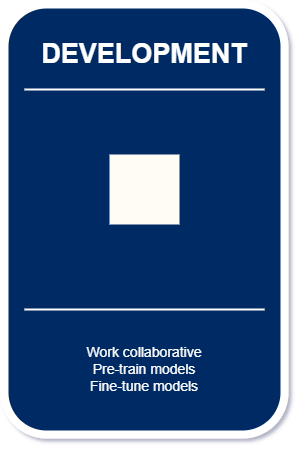
This stage involves experimenting with Generative AI models to deliver targeted outcomes. This involves choosing between open-source or commercial foundational deep learning models. The choice must consider things such as privacy, quality, latency and cost. Once the choices have been made, engineers will now consider the best solution in terms which models to fine-tune or pre-train, whether or not to adopt Retrieval-Augmented Generation for some of the models, and which model chaining system to use to increase efficiency and speed up responses.
How Databricks Helps
Access to pre-trained Generative AI models like Dolly and Hugging Face saves time and resources.
In terms of computational power needed to train models, Databricks offers managed clusters that support efficient large-scale model training.
Databricks’ AutoML feature simplifies experimentation, enabling data engineers and business analysts to participate in model development.
In the retail case, Databricks enables fine-tuning language models for personalized marketing messages and training time-series models for demand forecasting.
Validation: Ensuring Accuracy and Relevance
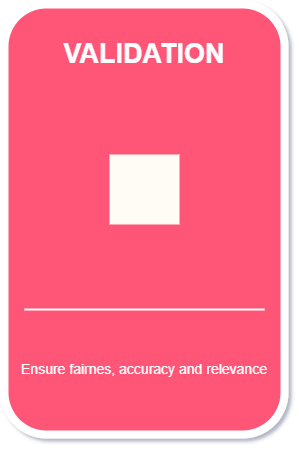
The development of generative AI has some drawbacks which when not managed properly can lead to bias or hallucinations in responses or output. Hence, generative AI models need to be validated for accuracy, fairness, and business relevance before deployment.
How Databricks Helps:
Tools like MLflow help track experiments and ensure reproducibility of outputs.
Advanced analytics and visualization dashboards validate model performance in terms of speed and accuracy of responses.
Databricks also offers built-in testing frameworks which help with identifying biases or anomalies in models.
In the business case under consideration, the retail team can validate marketing predictions against historical campaign results and ensure forecasting models account for regional and seasonal variability.
Deployment: Bringing Models to Life
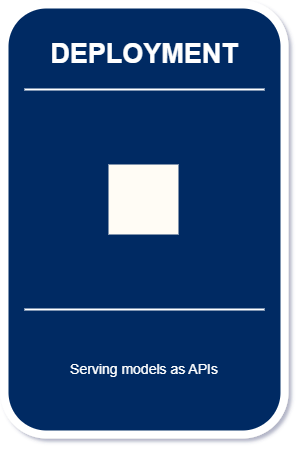
After validation, models must be deployed into production seamlessly. Businesses have a variety of ways to want to use these production-ready models. One common way is to develop application programming interfaces (APIs) which helps to integrate the solutions within already existing applications, such as an e-commerce application.
How Databricks Helps
Model deployment via Databricks integrates with APIs, making it easy to embed Generative AI into business workflows.
Real-time inference capabilities of Databricks’ platform power applications like dynamic marketing emails and chatbot interactions.
Scalability ensures that forecasting models handle large datasets across multiple regions.
Personalized offers can then be sent to customers through email and mobile apps, while demand forecasts help to optimize inventory orders in real time.
Monitoring and Iteration: Driving Continuous Improvement
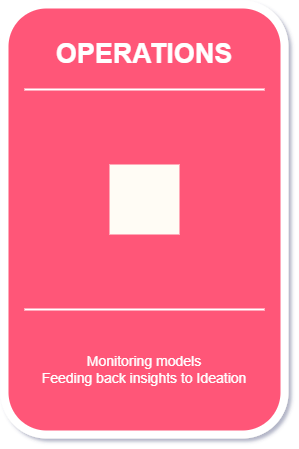
The work of continuously offering personalized and tailored solutions does not end with the deployment of the models. Generative AI adoption is a continuous journey. Models need regular updates and continuously improving the efficiencies of the system to adapt to new data and business goals. Also, insights from production data feed back into the ideation stage for new opportunities.
How Databricks Helps
Real-time monitoring ensures models perform as expected.
Databricks workflow feature can be used to automate the retraining of models using pipelines to keep models aligned with evolving business conditions.
The team from the retail company can use Databricks' monitoring tools to track campaign performance and inventory trends, iterating on models to improve future outcomes.
Conclusion

Adopting Generative AI may seem complex, but with the right tools and a clear roadmap, it becomes a transformative journey. Databricks provides the unified platform, scalability, and collaboration capabilities that businesses need to succeed at every stage.
For the retail company in this case study, Databricks enabled them to move from ideas to impactful outcomes, boosting sales and optimizing operations with Generative AI. Businesses across industries can achieve similar results by embracing the six stages of adoption and leveraging Databricks as their partner in innovation.